Descriptive and predictive analytics are essential tools for converting data into actionable insights. Descriptive analytics helps you understand what has happened by analyzing historical data, while predictive analytics forecasts future outcomes using advanced techniques. This article will guide you through understanding both, their techniques, tools, and real-world applications, helping you harness their power for better decision-making.
Key Takeaways
- Descriptive analytics focuses on summarizing historical data to provide insights into past performance, crucial for identifying trends and improving future strategies.
- Predictive analytics forecasts future outcomes by using historical data and advanced statistical techniques, enabling proactive decision-making in various business sectors.
- Integrating descriptive and predictive analytics enhances decision-making by providing a comprehensive view of past and future trends, allowing organizations to optimize strategies and resource allocation.
First step: understanding descriptive analytics
Descriptive analytics is the cornerstone of any data analysis strategy. It transforms raw data into meaningful insights, providing a clear view of what has happened in the past. Various techniques and tools in descriptive analytics empower businesses to make informed decisions based on historical data.
This foundational analysis is crucial for understanding trends, patterns, and anomalies that can inform future strategies and enhance overall business performance.
Definition and importance
Descriptive analytics is the simplest form of data analysis, focusing on summarizing historical data to interpret past events. Descriptive analysis provides a coherent overview of past data, allowing organizations to identify key factors that influenced outcomes and measure performance, including various types of data analysis.
Evaluating the effectiveness of past strategies and operations helps businesses identify areas for improvement and plan future strategies. This type of analysis helps businesses understand performance in context and interpret information accurately.
Techniques and tools
Descriptive analytics employs various techniques such as data aggregation, statistical methods, and types of data analytics to analyze historical data. Data aggregation involves collecting and organizing data into manageable sets. Data mining identifies patterns and trends within large datasets, enabling deeper insights.
Common tools used in descriptive analytics include Microsoft Excel, Power BI, Tableau, and Google Charts, which help visualize and interpret data effectively.
The real life: use cases and examples
Descriptive analytics is widely used across various industries to uncover hidden patterns and trends. For instance, in the retail sector, it analyzes historical sales data to determine customer purchasing trends. This information is invaluable for devising effective launch strategies and optimizing promotional efforts. In healthcare, descriptive analytics helps organizations identify spikes in emergency room admissions, guiding resource allocation and improving patient care.
Another compelling example is in sales, where descriptive analytics can reveal a seasonal surge in product demand, such as a video game console during the holiday season. Understanding these trends allows companies to better prepare inventory and marketing strategies.
The insights gained from descriptive analytics provide a solid foundation for making informed, data driven insights decisions that enhance overall business performance through key performance indicators kpis.
Exploring predictive analytics
While descriptive analytics focuses on understanding past events, predictive analytics takes it a step further by forecasting future outcomes. This type of analytics uses historical data and advanced statistical techniques to predict future events and trends. Applying predictive models to current data helps businesses anticipate customer behavior, optimize marketing strategies, and improve overall operational efficiency.
What is predictive analytics?
Predictive analytics leverages historical data and machine learning models to forecast future outcomes. Its primary goal is to predict likely future events based on past data. This provides a significant advantage to executives by enabling proactive, data-driven decision-making.
Predictive analytics can forecast various aspects such as customer behavior, purchasing patterns, sales trends, supply chain operations, and inventory demands. E-commerce companies analyze sales data and forecast product demand with predictive analytics, enabling them to optimize pricing during peak seasons.
Key techniques and algorithms
Key techniques in predictive analytics include regression analysis, decision trees, and neural networks. Many analytics platforms offer machine learning capabilities to enhance predictive modeling. These techniques play a crucial role in forecasting future trends and outcomes based on historical data.
Mastering these techniques and optimization algorithms is vital for effective decision-making in business environments.
Real-world applications and more
Predictive analytics is widely utilized across different sectors, showcasing its importance in modern business environments. In the healthcare sector, it enhances operational efficiency by predicting patient needs and reducing emergency hospitalizations. In finance, predictive analytics helps manage risks by refining credit scoring and improving investment strategies. These applications demonstrate how predictive analytics can lead to better decision-making and optimized operations.
Another real-world application is in marketing, where predictive analytics can anticipate customer behavior and preferences. Predicting what customers are likely to purchase enables businesses to tailor their marketing efforts to increase sales and customer satisfaction. The ability to forecast future outcomes based on historical data empowers businesses to stay ahead of trends and make strategic adjustments proactively.
Beyond the data: comparing descriptive and predictive analytics
Descriptive and predictive analytics serve different yet complementary roles in business. Descriptive analytics focuses on analyzing historical data to identify trends and patterns, while predictive analytics uses statistical modeling to forecast future outcomes, including descriptive predictive and prescriptive approaches.
Each type of analytics has its unique applications and benefits, and understanding their differences and similarities is crucial for leveraging their full potential in business strategies.
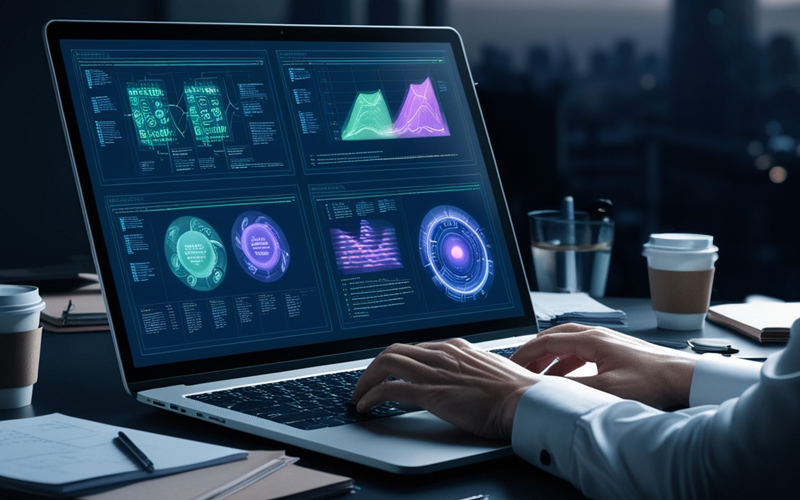
Differences and similarities
Descriptive analytics primarily provides insights into what has happened, whereas predictive analytics aims to predict what could happen based on historical data. Both types utilize data to derive insights, but their applications differ significantly.
Descriptive analytics focuses on historical analysis, while predictive analytics incorporates machine learning to enhance forecasting capabilities. Despite their different purposes, both can utilize statistical analysis techniques to provide valuable insights.
Complementary roles in business
Descriptive analytics provides organizations with insights into past performance. It also sheds light on customer behaviors and market dynamics. For example, in retail, it helps analyze historical sales data and customer purchasing patterns, which can be crucial for product launches. Recognizing different types of analytics helps businesses choose beneficial methods and drive their objectives. Descriptive analytics serves as a foundational layer that predictive analytics can build upon to make forecasts.
Integrating descriptive and predictive analytics provides businesses a comprehensive view of past performances and future forecasts. This integration enhances business intelligence and supports informed decision-making, ultimately empowering businesses to make data-driven decisions and stay competitive in the market.
Integrating descriptive and predictive analytics
Combining descriptive and predictive analytics can provide deeper insights and more informed decision-making in business operations. This integration allows businesses to gain a comprehensive understanding of past performance and future trends, enhancing their strategic decision-making.
Leveraging both types of analytics allows organizations to improve resource allocation and optimize their strategies.
Combining insights for strategy
Integrating insights from both descriptive and predictive analytics can lead to improved resource allocation and strategic planning. Using both types of analytics together enables businesses to identify trends and anticipate future market dynamics. Organizations need a formal strategy to effectively collect and analyze relevant data, avoiding decisions based on intuition alone.
Analyzing historical sales data and predicting future demand helps a business optimize its inventory levels and marketing efforts. This combination of insights not only enhances operational efficiency but also provides a competitive edge in the market.
The comprehensive view that both analytics types offer supports better decision-making and strategic adjustments.
Challenges and solutions in data analytics
Despite the benefits, implementing data analytics comes with its own set of challenges. From data quality issues to integration difficulties, businesses must navigate these obstacles to fully leverage the power of analytics.
Understanding these challenges and how to overcome them is essential for successful data analytics implementation.
Data quality and integration
Utilizing data validation and cleansing practices is essential for maintaining accurate and consistent data. Standardizing data formats early on can minimize cleansing efforts and facilitate better analysis. Top analytics software should offer robust data integration to connect various data sources. Effective analytics tools should integrate data management and analytical capabilities. Creating a centralized data repository can help organizations consolidate scattered data and improve accessibility for analytics. Understanding your organization’s data infrastructure is essential before selecting a data analytics tool.
Establishing strong access controls is necessary to secure data and ensure compliance with privacy regulations. Addressing data quality and integration challenges ensures businesses have reliable data for informed decisions and advancing their analytics initiatives.
Overcoming analytical roadblocks
Manual data collection is a common roadblock in analytics that can impede streamlined processes. It is time-consuming, not repeatable, and can cause data consistency issues. To overcome this, businesses should invest in automated data collection and integration tools that ensure data accuracy and consistency.
Streamlining data collection processes allows organizations to focus on deriving valuable insights and making data-driven decisions.
Leveraging data analytics tools
To fully harness the power of data analytics, businesses must leverage the right tools. Data analytics tools are becoming crucial for organizations to derive insights from vast data and support decision-making processes.
These tools offer features such as real-time data processing, visualization capabilities, and predictive modeling, which enhance the capabilities of both descriptive and predictive analytics.
Software solutions
Data analytics software solutions are essential for organizations looking to derive insights from large datasets and improve decision-making. An effective data analytics solution integrates analytics and data management capabilities for streamlined operations.
Cloud-based platforms provide on-premises and hybrid data access for fast insights. Leading software can process vast amounts of data quickly, facilitating real-time decision-making. User-friendly AI algorithms are transforming the generation of insights, making complex analyses more accessible.
Visualization tools like Tableau enable users to create engaging and informative data representations without extensive design skills.
Choosing the right tool
When selecting a data analytics tool, it’s essential to assess the scalability to match business growth. Selecting the appropriate tool is vital for aligning with business needs and ensuring effective resource utilization. Businesses should consider factors such as ease of use, integration capabilities, and the specific needs of their analytics projects. Choosing the right tool maximizes the value of data analytics investments and drives better business outcomes.
The right tools not only support the implementation of descriptive and predictive analytics but also pave the way for advanced analytics capabilities such as prescriptive analytics. This ensures that businesses are equipped to handle current data challenges and future trends effectively.
Future trends in data analytics
As technology continues to evolve, so does the field of data analytics. Companies are increasingly relying on emerging technologies to enhance their analytical capabilities and adapt to fast-changing environments.
Understanding future trends in business analytics and data analytics is crucial for businesses to stay competitive and leverage new opportunities that happen in the future.
Innovations in predictive modeling
Recent innovations in predictive modeling have significantly enhanced the capabilities of businesses to forecast and make decisions based on data. Machine learning models are now capable of identifying anomalies in data and alerting teams in real-time without human intervention. These advancements enable businesses to proactively adjust their strategies in response to emerging trends and anomalies.
Adopting innovative predictive modeling techniques enhances decision-making processes and improves overall business outcomes.
The growing role of prescriptive analytics
Prescriptive analytics is gaining prominence as it provides actionable insights that recommend optimal courses of action for businesses. It tells businesses what should be done to maximize their outcomes based on available data. The role of prescriptive analytics in decision-making is to help determine the best possible actions to take. The model utilizes data from different sources like statistics, machine learning, and data mining, including predictive prescriptive and diagnostic analytics. This helps in identifying potential future outcomes and determining the best actions for achieving desired results.
Effective prescriptive analytics requires large amounts of data to provide accurate and actionable insights. The use of machine learning in prescriptive analytics significantly reduces human error in decision-making processes, making it a valuable prescriptive analytics tool.
By leveraging predictive and prescriptive analytics, businesses can improve production, enhance customer experience, and drive growth. This type of analytics also anticipates what, when, and why certain business outcomes might occur, allowing for better planning and strategic execution.
In today’s data-driven world, mastering descriptive and predictive analytics is essential for making informed and strategic business decisions. Descriptive analytics provides a clear view of past performance, helping businesses understand what has happened and why. Predictive analytics takes it a step further by forecasting future outcomes, enabling companies to anticipate trends and make proactive decisions. The integration of both analytics types offers a comprehensive view that enhances business intelligence and supports better decision-making.
As we move forward, the importance of leveraging advanced analytics tools and technologies cannot be overstated. By understanding and applying these analytics types, businesses can optimize their operations, improve customer satisfaction, and achieve sustainable growth. The future of data analytics holds immense potential, and staying ahead of trends will empower businesses to thrive in a competitive landscape. Embrace the power of data, and let it guide your path to success.
Frequently Asked Questions
What is the main difference between descriptive and predictive analytics?
The main difference between descriptive and predictive analytics lies in their objectives; descriptive analytics aims to summarize and analyze past data to understand historical trends, while predictive analytics seeks to forecast future events based on statistical models and historical data.
How can businesses benefit from integrating descriptive and predictive analytics?
Businesses can significantly benefit from integrating descriptive and predictive analytics as it enables them to gain insights from past performance while forecasting future trends, which ultimately enhances strategic decision-making and optimizes resource allocation. For more personalized information, please
contact us here.
What are some common tools used in descriptive analytics?
Descriptive analytics commonly utilizes tools such as Microsoft Excel, Power BI, Tableau, and Google Charts, which facilitate data visualization and interpretation. These tools are essential for transforming raw data into meaningful insights.
What are the key techniques used in predictive analytics?
The key techniques in predictive analytics are regression analysis, decision trees, neural networks, and machine learning algorithms, all of which are essential for forecasting future trends and outcomes effectively. Understanding and applying these methods can significantly enhance decision-making processes.
What role does prescriptive analytics play in business decision-making?
Prescriptive analytics is critical in business decision-making as it offers actionable insights and recommendations for optimal actions based on data analysis, enabling organizations to achieve their desired outcomes effectively. By leveraging prescriptive analytics, businesses can make informed decisions that align with their strategic objectives.
0 Comments